Panasonic Holdings Corporation announced the acceptance of papers to the European Conference on Computer Vision (ECCV) 2022, one of the top international conferences in AI and Computer Vision, and the IEEE/RSJ International Conference on Intelligent Robots and Systems (IROS) 2022, one of the top international conferences in AI and Robotics. Authors and co-authors will make presentations at the conferences from October 23 to 27, 2022.
Panasonic Holdings strives to deliver innovative products and services that are able to solve social issues as well as those in lifestyle by implementing AI technology into Panasonic’s key business areas: home appliances, housing, automotive devices, and B2B solutions. Furthermore, we encourage our team to publish our latest research findings to international conferences in order to actively contribute to the academic and industrial AI research communities.
Multiple research projects jointly conducted by our teams and collaborators have recently received international recognition in computer vision and robotics, two fields that are essential to the social implementation of AI.
Panasonic Holdings will continue to work with researchers inside and outside the company to advance the research and development of AI technology that contributes to the happiness of our customers through our products and services.
– About ECCV and IROS
ECCV is one of the top conferences in the fields of AI and computer vision, along with CVPRÂ [1]Â and ICCVÂ [2]. Known as a place for AI researchers around the world to discuss their latest achievements, the acceptance rate is as narrow as 20% to 30%.
List of research accepted to ECCV:
1. Rethinking Generic Camera Models for Deep Single Image Camera Calibration to Recover Rotation and Fisheye Distortion
2. MTTrans: Cross-Domain Object Detection with Mean-Teacher Transformer
3. Equivariance and Invariance Inductive Bias for Learning from Insufficient Data
IROS is one of the top conferences in the fields of AI and robotics, along with ICRAÂ [3]. It is known as a place for AI and robotics researchers around the world to discuss their latest achievements beyond their individual research areas.
List of research accepted to IROS:
4. DreamingV2: Reinforcement Learning with Discrete World Models without Reconstruction
Research Details:
1. Rethinking Generic Camera Models for Deep Single Image Camera Calibration to Recover Rotation and Fisheye Distortion
Background:
Fisheye cameras, with their large fields of view, are expected to be used in various applications, such as wide-view surveillance and obstacle detection. By contrast, the large fisheye image distortion prevents the reuse of existing recognition models. Therefore, it is necessary to convert these images into ones with less distortion using camera calibration. Conventional calibration methods have two problems: large time-consuming and requirements of specific measurement systems. To address these problems, we have researched a calibration method that is easy to use and has high accuracy for largely distorted images.
Abstract:
We introduced a calibration method that estimates distortion and camera rotation from a single image using deep learning. To achieve precise calibration for fisheye cameras, we proposed a geometric camera model with high accuracy and a small number of parameters. Although we train only a model, this designed model enables high calibration accuracy from a single image. Furthermore, optimal loss-function parameters can be determined before training through numerical simulations instead of trials and errors. This simulation approach leads to the improvement of calibration accuracy and the reduction of training time. Our paper was accepted on the basis of the verification of large-scale datasets and several actual cameras, and the achievement of state-of-the-art performance [4].
Future Outlook:
Although various camera applications are used daily, few people know that the operation is time-consuming. Our method can reduce the enormous amount of labor by rectifying image distortion using only an image. We expect our method to be widely used for image pre-processing because of the instant recalibration for the stable operation of camera applications. In particular, our method is suitable for applications, such as ADAS and sensing on drone cameras because we assume that these cameras are largely affected by vibration and collision.
Thorough research in image processing and cutting-edge AI has enabled us to propose our method based on both camera geometry and deep learning. We are continuously studying advanced technology development along with the knowledge described above.
This research is the joint work of Dr. Nobuhiko Wakai, Platform Division, Panasonic Holdings, Mr. Satoshi Sato and Mr. Yasunori Ishii, Technology Division, Panasonic Holdings, and Prof. Takayoshi Yamashita, Chubu University.
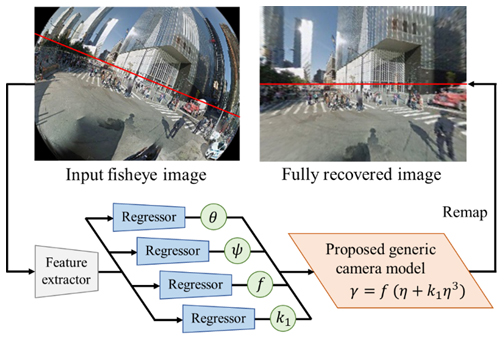
2. MTTrans: Cross-Domain Object Detection with Mean-Teacher Transformer
Recent object detection models have achieved promising performance under a similar domain as train dataset. However, they are inaccurate under different environments and require large-scale labeled data in order to overcome such environment shift.
Abstract:
This paper proposed an object detection model applied to new different environments without annotation labels. Concretely, we use pseudo labels instead of annotation labels to train models. For accurate pseudo labels, we proposed multi-level feature alignments, which suppress discrepancy of feature representations among domains. We evaluate the robustness of our model towards domain shift with public datasets and confirmed that our proposed method achieves state-of-the-art performance in this field [5].
Future Outlook:
Our contribution is expected to reduce costs and improve robustness when adapting object detection models into different domain for recognition functions in products such as industry robots and cars. We will continue to develop new technology by jointly working with universities and applying new technology to industrial applications.
This research is the joint work of University of California, Berkeley AI Research (BAIR), Peking University, Beihang University, Mr. Denis Gudovskiy, Panasonic R&D Company of America AI Laboratory, Dr. Yohei Nakata, and Mr. Tomoyuki Okuno, Technology Division, Panasonic Holdings.
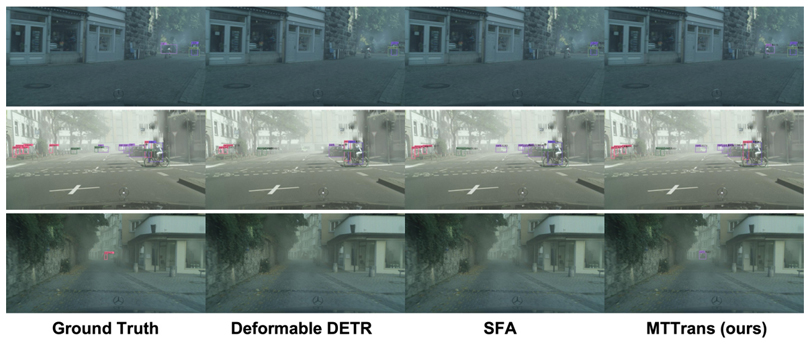
3. Equivariance and Invariance Inductive Bias for Learning from Insufficient Data
Background:
Deep learning from insufficient training data is challenging. Besides the common motivation that collecting data is expensive, we believe that how to narrow the performance gap between insufficient and sufficient data is the key challenge in machine learning model generalization—even if the training data is sufficient, the testing data can still be out of the training distribution. Thus, our focus is to train deep models with insufficient data without using external data.
Abstract:
Deep learning from insufficient data negatively affects generalization. The crux of improving the generalization of insufficient data is to recover the missing class feature while removing the “contextual” environmental feature. To this end, we propose two inductive biases to guide the learning: equivariance for class preservation and invariance for environment removal. Equivariance for class preservation is obtained with an off-the-shelf contrastive-based self-supervised feature learning approach, whereas, for environmental invariance, class-wise invariant risk minimization (IRM) is used.
Future Outlook:
Learning from insufficient data is inherently more challenging than that from sufficient data—the latter will be inevitably biased to the limited environmental diversity. We will challenge further narrowing down the performance gap with collaborators to tackle the dataset-related issues that are essential for the social implementation of AI.
This research is the joint work of Nanyang Technological University, Singapore Management University, Panasonic R&D Center Singapore.
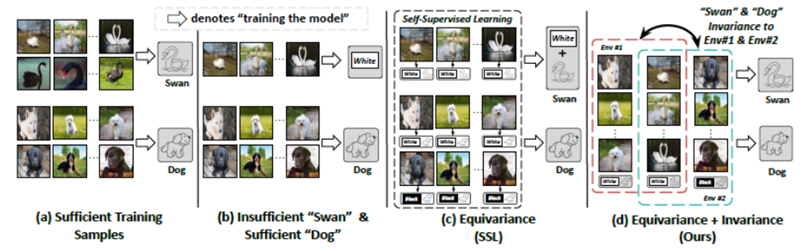
4. DreamingV2: Reinforcement Learning with Discrete World Models without Reconstruction
Background:
Reinforcement learning based on world models [6] has been rapidly studied in recent years and is expected to have promising applications such as visual servoing of industrial robots. On the other hand, in the cases that the objects to be controlled and manipulated are small, reinforcement learning would fail since the robots cannot recognize the objects.
Abstract:
This paper proposed DreamingV2, which realizes visual models that can recognize small objects by applying self-supervised learning originally proposed in the computer vision field. Furthermore, by making discrete variables called latent states inside the world models, DreamingV2 realizes kinematic models that can capture discontinuous dynamics including robot-object contact. Simulation experiments have demonstrated that DreamingV2 can achieve the world’s highest performance [7] in various simulated robot tasks, such as picking up objects with a robot arm.
Future Outlook:
Our contribution is expected to replace manual labor in factories with robots. In addition, it will reduce programming and tuning for industrial robots, which currently requires a lot of time and labor. We will continue to develop this technology for industrial applications in factories, while proceeding with evaluation using real robots.
This research is the joint work of Dr. Masashi Okada, Technology Division, Panasonic Holdings, and Prof. Tadahiro Taniguchi, Ritsumeikan University.
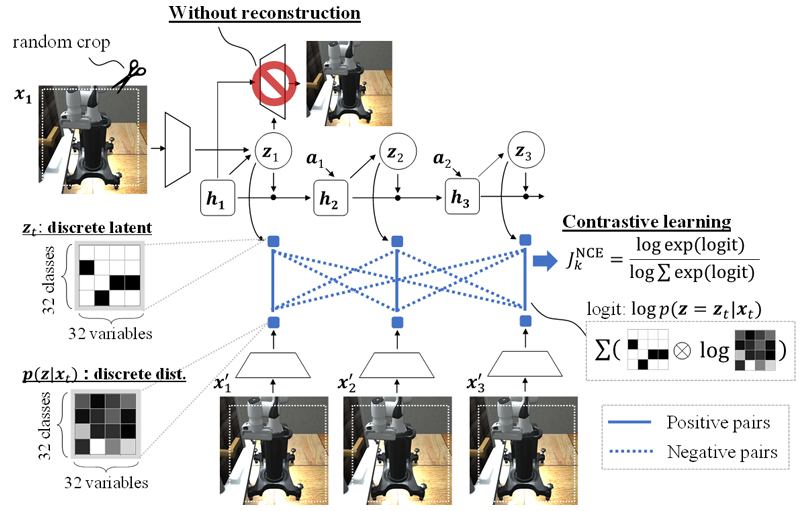
Apart from the four research papers mentioned in this press release, one research paper conducted in collaboration with Panasonic Connect Co, Ltd. was also accepted to IROS2022: “Tactile-Sensitive NewtonianVAE for High-Accuracy Industrial Connector Insertion”
The paper below was also accepted to the ECCV workshop, which will be held in conjunction with ECCV2022.
“Invisible-to-Visible: Privacy-Aware Human Segmentation using Airborne Ultrasound via Collaborative Learning Probabilistic U-Net”